报告人1:
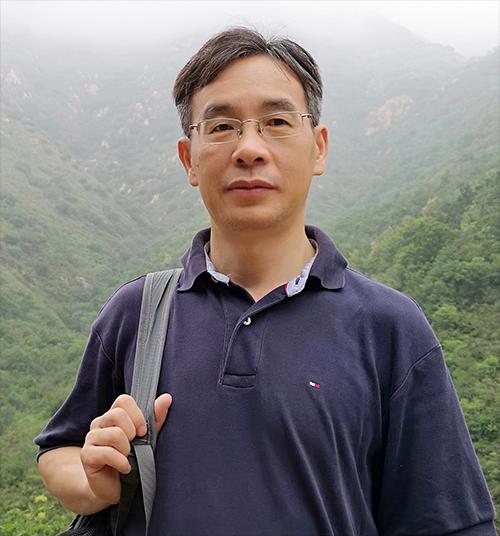
方勇纯 南开大学,教授, 博导,
国家杰青,长江学者
Biography
方勇纯,南开大学教授,博士生导师,研究生院常务副院长,双一流办主任。分别于1996年和1999年获得浙江大学本科和硕士学位,2002年获美国克莱姆森大学博士学位,2002年至2003年在美国康奈尔大学进行博士后研究,2003年至今,任教于南开大学。2013年获得国家自然科学基金杰出青年基金资助,2017年入选教育部长江学者特聘教授,2019年,入选国家百千万人才工程,2020年,获国务院政府特殊津贴。
Title: 仿生蛇形机器人轨迹规划与控制
Abstract:与其它机器人相比,蛇形机器人可以在狭窄管道等环境中作业,因此具有广阔的应用前景。轨迹规划与控制是蛇形机器人高效作业的关键步骤。本讲座主要基于学习等方法,讨论蛇形机器人的轨迹规划与控制问题,具体包括:基于深度模仿学习的轨迹规划,基于强化学习的步态优化,基于强化学习的跟踪控制,基于管道半径估计的爬升控制,等等。
报告人2:
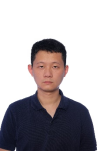
徐 翔 南方科技大学,研究员,博导,
国家优青(海外)
Biography
Xiang Xu received the Bachelor of Engineering degree from Nanjing University of Science and Technology, China in 2014 and the Ph.D. degree from City University of Hong Kong, Hong Kong in 2018. From 2018 to 2021, he was a postdoctoral fellow in the Department of Biomedical Engineering, City University of Hong Kong. He is now an associate professor in the School of System Design and Intelligent Manufacturing, Southern University of Science and Technology, Shenzhen, China. His research interests include multi-agent systems, time delay systems, nonlinear control and boundary control of PDEs.
Title:FAS Models for Strict-Feedback Systems with Nonuniform Dimensions
Abstract: This talk extends the existing works on fully-actuated system (FAS) models for strict-feedback systems (SFSs). We consider control problems for SFSs with nonuniform dimensions. In particular, the subsystems in the considered SFSs are assumed to have increasing dimensions, which is different from and also more complex than the commonly-considered SFSs where the subsystems have the same dimension. The systems are transformed into FAS models, based on which the stabilizing controllers can be directly given. Besides first-order SFSs, second-order and high-order SFSs are also considered.
报告人3:
赵春晖 浙江大学求是特聘教授、博导
国家杰青
Biography
Chunhui Zhao, Qiushi Distinguished Professor at Zhejiang University. Her research interests include statistical machine learning and data mining for industrial application. She has authored or coauthored more than 190 papers in peer-reviewed international journals. She has published 3 monographs and one national textbook. She authorized more than 60 invention patents. She is principal investigator of a Distinguished Young Scholar Program supported by the Natural Science Foundation of China. She has hosted more than 20 scientific research projects, including the NSFC funds, National key R&D project, provincial projects and corporate cooperation projects. She has received the Ministry of Education Natural Science Award and other provincial and ministerial awards. She also received more than ten academic awards, including the First Prize of Natural Science of Chinese Association of Automation, the First Young Women Scientists Award of Chinese Association of Automation, etc. She has served senior editor of Journal of Process Control, associate editors of Control Engineering Practice and Neurocomputing, etc.
Title: Theoretical exploration and practice of industrial process fault diagnosis based on zero sample learning
Abstract: Fault diagnosis system is an important guarantee for the safe and reliable operation of industrial processes. Data-driven fault diagnosis modeling often depends on the collected sufficient historical fault data. However, in actual industrial processes, it is common that process failures have no historical samples and no labels. In this regard, we need to deal with a very challenging fault diagnosis task, that is, to consider diagnosing when there are no historical fault samples available for model training. We introduced the concept of zero-shot learning into the industrial field for the first time, and innovatively established a zero-shot-learning fault diagnosis method. By skillfully introducing a priori modeling knowledge with fault description as the carrier and adopting the attribute migration method, we overcame the concerned bottleneck problem that traditional fault diagnosis research cannot meet the sample size constraint. The fault diagnosis effects in the case of zero samples are verified in the real megawatt thermal power process, and the results show that it is feasible to diagnose unseen target fault without samples. On this basis, the existing challenges, difficulties and future research directions are revealed.
报告人4:
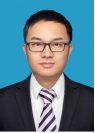
那 靖 昆明理工大学,教授,博导,
长江学者特聘教授
Biography
Jing Na is currently a Professor with the Faculty of Electrical & Mechanical Engineering at Kunming University of Science & Technology. He received the B.S. and Ph.D. degrees from the School of Automation, Beijing Institute of Technology, China, in 2004 and 2010, respectively. From 2011 to 2012, he was a Monaco/ITER Postdoctoral Fellow with the ITER Organization, France. From 2011 to 2012, he was a Marie Curie Fellow with the University of Bristol, UK. He has hold also visiting positions with the Universitat Politecnica de Catalunya, Spain, and with the University of Bristol, UK.
His current research interests include parameter estimation, adaptive control, and nonlinear control with application to vehicle systems, servo mechanisms and energy conversion plants (e.g., engine, wave energy convertors, etc.). He has authored/co-authored more than 100 peer reviewed journal and conference papers. Dr Na has been awarded the Best Application Paper Award of the 3rd IFAC International Conference on Intelligent Control and Automation Science (IFAC ICONS 2013), and the 2017 Hsue-shen Tsien Paper Award.
He is currently an Associate Editor of the IEEE Transactions on Industrial Engineering, and Neurocomputing. He has also served as an international program committee Chair of CCDC 2021 and DDCLS 2019 and IPC member of many international conferences. He has organized several special issues in international journals, and invited session in several prestigious conferences.
Title: Adaptive Parameter Estimation and Control via Estimation Error: Theory and Application
Abstract: Adaptive parameter estimation and adaptive control have been well developed to achieve modeling and control for uncertain systems. However, the well-known parameter estimation and adaptive control methods have been mainly designed based on the gradient algorithms (with appropriate modifications) by using the prediction error or control error. Hence, the parameter estimation convergence and the online verification of the required persistent excitation (PE) condition are generally difficult with this framework. In this talk, we will introduce a novel robust, fast adaptive parameter estimation framework, where the estimation error between the unknown parameters and their estimates are explicitly obtained and then use to drive online adaptation algorithms. This new adaptation even allows to achieve finite-time parameter estimation, and can be easily incorporated into adaptive control designs to achieve tracking control and parameter estimation simultaneously. We will also introduce an intuitive and numerically feasible approach to online verify the PE condition. Finally, several practical application of this new adaptation to in-car parameters estimation, ITER cryogenics system identification, and adaptive control for robotics, wave energy converters (WECs) and other realistic systems will be presented.